Another Bit of Pandemic Fallout: The Weaponization of Absolute Risk Statistics – Absolutely Maybe – PLOS
Back in the good old days, we only had to tear our hair out when people used relative risks without context, thereby giving an exaggerated impression of treatment effectiveness. That’s when a negligible difference in risks is trumpeted with the classic, “drug x halved the risk“.
At least even then the relative risk is still a very useful bit of information: you can use it to estimate the potential impact on people at very different starting levels of risk. And it can help people get larger potential benefits and harms into perspective. Now, though, the menace of their misuse has been joined by a trend that’s far more pernicious: using absolute risk statistics to try to dramatically minimize the value of vaccines. It wasn’t a standard go-to of anti-vax campaigning in the past. But it’s making so many appearances now, that I decided it was definitely time to update the way I talk about risk communication – and this old cartoon:
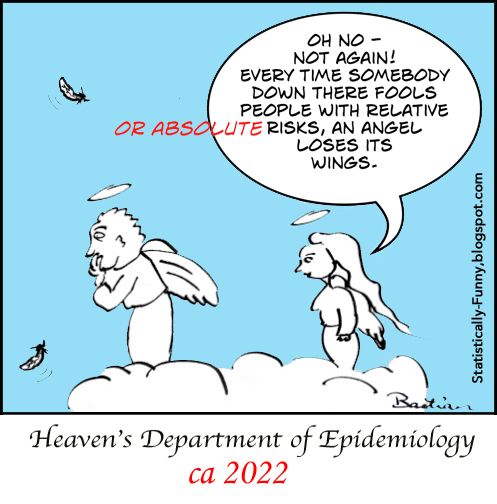
This new wave of rhetoric kicked into gear soon after the announcement of the Covid mRNA vaccines’ super high early efficacy. It was one of a swathe of doubt-sowing arguments Peter Doshi included in an opinion piece at the BMJ in January 2021. His piece topped their most-read list for a while, and it was amplified at Robert F. Kennedy Jr’s anti-vax website. (I wrote a debunking of Doshi’s 6 major points here.)
It was followed a few weeks later by another opinion piece making the absolute risks case from Ronald B. Brown, who added a further absolute risk statistic to the argument – number needed to vaccinate (NNV). In April, Piero Olliaro and colleagues got on the bandwagon at Lancet Microbe. Fact-checkers tackled the resulting spray of anti-Covid-vax misinformation that piece launched, including at Instagram. Statisticians and others responded with rebuttals, too – Andrew Althouse at Medscape, Luis C.L. Correia and Denise Matias replied to Lancet Microbe, and Stephen J. Senn blogged.
Nevertheless, this claim took off: that, according to “The Lancet”, absolute risk statistics prove that Covid vaccines don’t actually work – because they show the “real” efficacy rate, and the standard vaccine efficacy rate based on relative risk is a statistical sleight of hand by pro-vaxers to hide this from the general public. It has settled in as an anti-vax “Gotcha!” claim and conspiracy theory rolled into one. I’m concerned the opinion pieces in journals could also spread this thinking more widely.
The absolute risk argument is just tailor-made for this misuse – it’s so technical, that using the term and flinging the numbers around provides the illusion of superior scientific knowledge, while simultaneously being hard to counter in a quick unrehearsed few words on the fly. Another person tweeting it at me this week as if it was a slam dunk argument triggered this post: I wanted an accessible detailed post at the ready for all the next times.
Let’s start with a quick overview of the 3 statistics:
- Absolute risk reduction (ARR) is also called the risk difference (RD): One risk is subtracted from the other.
- For example, if the risk in an untreated group is 40%, and it’s 10% in the treated group, the ARR is 30% (40 minus 10) – the risk dropped 30 percentage points.
- Relative risk reduction (RRR): One risk is divided by the other. The standard vaccine efficacy statistic is a relative risk reduction.
- In our example, the RRR is 75% – 30 is three-quarters of 40.
- Number needed to vaccinate (NNV): This is the vaccine version of the number needed to treat, using the absolute risk to calculate how many people need to use the vaccine to prevent a single case. (It’s the inverse of the ARR: 1 divided by the ARR.)
- In our example where the ARR is 30%, the NNT/NNV is 3.
Although these statistics are all calculated with the same data from vaccine trials, that doesn’t mean they’re interchangeable or equally informative. The RRR is the statistic we need because it should be a reliable estimate of how much the vaccine could reduce the risk if we are exposed to the virus.
The absolute numbers, on the other hand, vary depending on how much virus circulates in the early part of the trial, and how long the efficacy evaluation continued. The absolute number of people getting sick in a short space of time is going to be small, while the potential risk of getting sick in a long-term epidemic goes on, climbing all the while during surges.
Here’s a timeline of the big Pfizer trial to show how short the time was, and how the risk of being exposed was climbing for the people in it:
- End of July 2020: Upcoming start of the phase 2/3 trial announced. It took a month for each new recruit to reach the point where efficacy starts to count (2 shots 3 weeks apart, plus 7 days).
- End of October 2020: The trial was fully recruited, but somewhere around 6,000 participants still hadn’t had their second shot.
- November 9, 2020: Results of the first interim efficacy analysis were announced. With a data cut-off of November 4, it had only been around 3 months since the first participant got their first shot. At that point, 92 trial participants had had symptomatic Covid.
- December 2020: The journal article for the trial was published. At what appears to be November 14, the number of trial participants who had had symptomatic Covid was 170.
Only a small proportion of the trial participants would even have been in harm’s way of the virus in only 3 months – but the chances were it wouldn’t stay that way, especially when non-vaccine precautions petered out. Risk of exposure rises and falls over time, and depending on context.
Take me, for example. I live in the country in Australia. At the time I initially got vaccinated in 2021, it was still Zero Covid around here, and precautions were strict to keep it that way. I was basically at zero risk of getting infected if I stayed in my little town. But if I’d gotten on a plane to London late in the year, my risk would have shot up overnight, and there would be a meaningful chance that the vaccine would be standing between me and potentially dangerous illness. And now we’re going into winter here with a lot of Covid circulating and very few precautions in place: my 3 vaccine shots have heavy lifting to do.
The trials were designed to get an answer on relative risk as soon as possible, so the number of “events” (symptomatic Covid) was pre-specified at as low a number as you could to reliably estimate a range for the RRR. That has to be taken into account too, as Althouse explained in his rebuttal of the absolute risk arguments: “With event-driven trial design, the ARR is ‘bounded’; it literally cannot be higher than the number of events at which the analyses are scheduled, divided by the number of patients in the placebo arm”.
Consider a trial that waited for a larger number to accrue than the Pfizer trial: the one for the J&J vax. The number of events required for its first analysis was 154, and the number of events in the final analysis was 1,551, compared to Pfizer’s 170. In the Lancet Microbe piece advocating absolute risk statistics, Olliaro and colleagues reckoned that going by NNV, a single dose of the J&J vax had higher efficacy against symptomatic disease than 2 Pfizer doses. It was in fact less effective for that outcome in rollout, just as the RRRs of the trials showed. The absolute risk statistics they were arguing would improve how well-informed we were, didn’t do that. They were misleading, and that’s no surprise. Whereas results on rollout were consistent with the trials’ vaccine efficacy rates.
Olliaro and colleagues wrote, “ARRs tend to be ignored because they give a much less impressive effect size than RRRs”. That’s not true. When it comes to vaccine trials for infectious diseases, RRRs are used because that makes more sense.
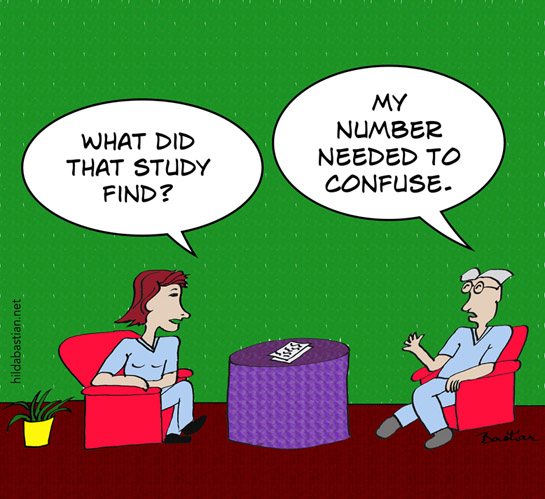
~~~~
Disclosures: I’m a long-time critic of NNTs, see here and here. My interest in Covid-19 vaccine trials is as a person worried about the virus, as my son is immunocompromised: I have no financial or professional interest in the vaccines. I have worked for an institute of the NIH in the past, but not the one working on vaccines (NIAID). More about me.
The cartoons are my own (CC BY-NC-ND license). (More cartoons at Statistically Funny.)
This article has been archived for your research. Find the original article here.