Understanding the spread of COVID‐19 misinformation on social media: The effects of topics and a political leader’s nudge
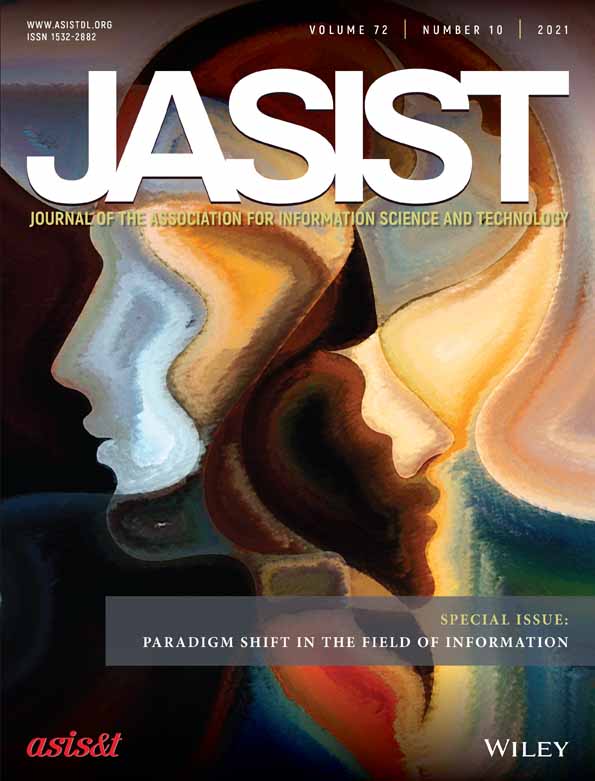
2.1 The detection and diffusion of misinformation
Previous studies on misinformation have focused on two tasks: misinformation detection (Kumar & Geethakumari, 2014; Shu et al., 2017; Zhang et al., 2018) and misinformation diffusion (Allcott & Gentzkow, 2017; Fung et al., 2016; Garrett, 2019; Grinberg et al., 2019; Mosleh et al., 2020; Wood, 2018).
Misinformation detection is usually a binary classification task (misinformation/true) or a multi-class classification task (true/mostly true/half true/mostly false/false) (Sharma et al., 2019). Most existing studies have leveraged the content or context of information (Wu et al., 2019; Zhou & Zafarani, 2018). (a) Content-based methods mainly include knowledge-based and style-based detections. Knowledge-based detections evaluate the reliability of content, such as evaluating whether the knowledge from text content is false via manual fact-checking (e.g., expert-based, or crowd-sourced fact-checking) (Grinberg et al., 2019) or automatic fact-checking through Natural Language Processing. Style-based detections focus on writing styles. For instance, misinformation usually includes shocking images, skeptical headlines, and exaggerated language to attract people’s attention (Baptista & Gradim, 2020). (b) Context-based detections mainly investigate information propagation patterns and the credibility of its sources. Propagation-based approach focuses on how misinformation spreads. Epidemic models, cascade models, and generative models have been adopted to characterize misinformation patterns and reduce the process of spreading (Bak-Coleman et al., 2021; Cinelli et al., 2020; Tambuscio et al., 2015). Researchers have revealed that misinformation spreads faster, deeper, and broader than trusted information (Del Vicario et al., 2016; Vosoughi et al., 2018). Credibility-based detections examine the credibility of news sources by assessing those who create and spread the news (e.g., social media accounts) (Shu et al., 2017).
The spread of misinformation can be attributed to the lack of “third-party filtering, fact-checking, or editorial judgement” on the internet (Ali & Zain-ul-abdin, 2021; Allcott & Gentzkow, 2017; Ennals et al., 2010), including on social media. People believe in and share misinformation for individual and social reasons (Nahon & Hemsley, 2013; Sharma et al., 2019). At the individual level, many people lack the ability to recognize misinformation, especially citizens with lower levels of education (Allen et al., 2020; Bessi et al., 2015; Georgiou et al., 2020; Grinberg et al., 2019; Guess et al., 2019). On the contrary, people with higher education and spend more time on media are more likely to discern misinformation (Allcott & Gentzkow, 2017). At the social level, social networking and interactions enabled by social media platform make it easier for a piece of information to “go viral” (Nahon & Hemsley, 2013; Galuba et al., 2010). During the diffusion process on social media, a group of “influencers” can have the potential power to influence a disproportionately large group of people (Weimann, 1994). Many studies have attempted to identify such influential users on social media and quantify their influence via retweets, follower count, network centralities, or sentiment dynamics (Cha et al., 2010; Dubois & Gaffney, 2014; Kwak et al., 2010; Zhao et al., 2014). Nevertheless, for the spread of political information, having more followers does not necessarily mean a higher level of influence (Hemsley, 2019).
The COVID-19 pandemic became a major event, for which misinformation was prevalent. One reason is that people are more likely to share misinformation during crises (e.g., natural disasters, wars, terrorist attacks, virus breakouts) (Mosleh et al., 2020; Van Prooijen & Douglas, 2017; Vosoughi et al., 2018). Other reasons include lower trust in science (Plohl & Musil, 2020; Roozenbeek et al., 2020), a lack of analytical thinking (Roozenbeek et al., 2020), and the emergence of purposely designed misinformation articles (Cinelli et al., 2020; Ordun et al., 2020; Sharma et al., 2020). Existing studies of misinformation about COVID-19 focused on descriptive analysis, such as the sentiment, topics, and geographical distribution (Li et al., 2020; Sharma et al., 2020; Singh et al., 2020). Roozenbeek et al. (2020) characterized individual predictors of people’s susceptibility to COVID-19 misinformation using survey data, but the sample size is limited in scale. Therefore, this study represents the first effort to investigate the spread of misinformation about COVID-19 with a large-scale dataset.
However, the literature on misinformation spread has mainly studied misinformation about a much more specific topic, such as candidates of political elections (Allcott & Gentzkow, 2017; Garrett, 2019; Grinberg et al., 2019; Hemsley, 2019) or alternative treatments of diseases (Fung et al., 2016). In contrast, misinformation about COVID-19 covers a broader spectrum of topics, including politics (e.g., lock down measures), public health (e.g., 5G causing the virus), medicine (e.g., the use of hydroxychloroquine to treat COVID-19), vaccine (e.g., conspiracy stories against Bill Gates and Anthony Fauci), and so on (Singh et al., 2020). The spread of such multi-topic misinformation about COVID-19 has posed threats to people and our society in several ways. First, it undermines the credibility of scientific news and individual capacity (Hopf et al., 2019). Second, it has created confusion and misguided people’s behaviors in the fight against the virus (e.g., discouraging people from adopting precautions or wearing masks) (Roozenbeek et al., 2020; Tasnim et al., 2020). Third, it also leads to hatred, discrimination, and social unrests (Yusof et al., 2020). However, it remains unknown which COVID-19 topics are more likely to spread on social media. In addition, key influencers, including political leaders (Hahl et al., 2018), contributed heavily to the spread of misinformation (Wood, 2018; Zollo & Quattrociocchi, 2018). One such example is former US President Donald J. Trump, who has been criticized for using his tweeting “deflection” strategy to frame himself as the only reliable source of truth and erode the public trust on mainstream media outlets throughout the 2016 U.S. Presidential Election (Ross & Rivers, 2018). Despite their importance in sharing information with the public and people’s trust in them to provide accurate information during a crisis, political leaders’ effects on the spread of misinformation have not been studied with empirical data.
2.2 The HSM for misinformation spreading
Sharing information (e.g., retweeting) is a key feature provided by social media sites such as Twitter (Cha et al., 2010; Suh et al., 2010). People need to process information before sharing it (Engelmann et al., 2019). Therefore, we based our analysis of sharing misinformation on the HSM, which states that people process information using two modes—heuristic processing and systematic processing (Chaiken, 1980; Chen & Chaiken, 1999). The systematic processing mode mainly focuses on the content of a message and involves considerable cognitive effort to scrutinize, comprehend, and evaluate the validity of information (i.e., fully processing the content of the message). In contrast, heuristic processing requires less cognitive processing—people make quick decisions to adopt information based on mental shortcuts or rules of thumb that have been stored in their memory and can be reprocessed in a given situation, such as the readability and familiarity of information content (e.g., the ease to get and comprehend information), the credibility of information sources (e.g., relying on statements from experts and leaders they trust), or the crowd behaviors (e.g., supporting decisions endorsed by a large number of people) (Bargh, 1989; Bohner et al., 1995; Chaiken & Maheswaran, 1994; Chen et al., 1996; Chen & Chaiken, 1999; Lin et al., 2016; Wathen & Burkell, 2002).
Beyond processing information, empirical studies using the HSM found that both systematic processing and heuristic processing influence individual’s sharing of information (Xiao et al., 2018). Specifically in the context of information sharing in social media, systematic processing assesses a tweet’s content itself, while heuristic processing has been operationalized to focus on a tweet’s sources, others’ endorsement of the tweet, and its communication styles (Engelmann et al., 2019; Firdaus et al., 2018; Liu et al., 2012).
Another phenomenon related to heuristic processing is “nudge”—the indirect yet deliberate move to alter people’s choices or make people more subject to heuristic processing (Saghai, 2013; Schmidt, 2017; Thaler & Sunstein, 2009). Even though nudges usually occur in subtle and often unnoticeable ways, they have been effective in promoting individual behaviors such as those in taxation (Holz et al., 2020), education (Benhassine et al., 2015), healthcare (Blumenthal-Barby & Burroughs, 2012), and marketing (Tan et al., 2018). Specifically, nudges can influence people’s behaviors through two types of heuristics: familiarity heuristics and consensus heuristics. Familiarity heuristics indicate that individuals are more likely to make familiar decisions based on previous experiences (Tversky & Kahneman, 1974). For example, social media users are more likely to endorse information from repeated exposure to certain information (Ali & Zain-ul-abdin, 2021; Garcia-Marques & Mackie, 2001). In fact, even a single prior encounter can increase the believability of information (Pennycook et al., 2018). In contrast, consensus heuristics suggest that an individual’s behavior is an extension of other people’s behaviors (Chaiken, 1987; Kelley, 1967). For instance, people tend to conform to the judgment of a large number of people or even if the judgment is incorrect (Bond & Smith, 1996). Specifically, while directly sharing a piece of misinformation on social media would certainly help its spread, we would like to investigate whether influential individuals, such as political leaders, are also effective in nudging people’s sharing of misinformation with indirect approaches.
Specifically for COVID-19, it is difficult for citizens to evaluate the validity of information about COVID-19 during the pandemic, because of limited knowledge about this new virus, information overload, or anxiety (Li et al., 2020; Rathore & Farooq, 2020; Van Bavel et al., 2020). Such situations can increase people’s reliance on the heuristic mode (Eastin, 2001; Lang, 2000; Ratneshwar & Chaiken, 1991; Zuckerman & Chaiken, 1998) when they process and share information related to COVID-19.
As a result, many people turn to their political leaders during such a crisis and “rally around the flag” (Baekgaard et al., 2020; Hetherington & Nelson, 2003; Mueller, 1970), because they are confident in their leaders’ ability to handle challenges (Zhu et al., 2012). Studies suggested that some political leaders’ tweets about COVID-19 quickly became viral (Rufai & Bunce, 2020) and have influenced people’s judgment of the pandemic (Van Bavel et al., 2020).
Therefore, based on large-scale data of COVID-19 misinformation and the behaviors of Donald J. Trump on Twitter, this study attempts to address two specific research questions with the HSM framework.
RQ1.What are the effects of different topics of COVID-19 misinformation on the spread of such stories on social media?
RQ2.What roles did Donald J. Trump play in the spread of COVID-19 misinformation on Twitter?
*** This article has been archived for your research. The original version from Wiley can be found here ***